06 February 2024
12336
32 min
3.67
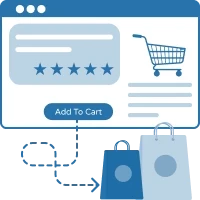
Personalized Product Recommendations: The Technology in Details and Case Studies
Turning curious browsers into confident buyers is a challenge in the ever-evolving world of ecommerce. Did you know that a staggering 80% of consumers are more likely to purchase after a personalized experience? Imagine if your virtual storefront resonated with each visitor on a personal level, leading them down a path to purchase that was relevant and compelling. This is the transformative potential of personalization that can significantly improve your business.
Even the pain of the ‘one-time sale’ is quelled by the power of personalization. Statistics show that 78% of consumers are more likely to repurchase from companies delivering a customer experience that matches their needs and expectations. This is the foundation for building the loyalty that drives business success. By the way, the same number of customers are more likely to recommend such a company to their family and friends.
Product recommendations are one of the most powerful tools for personalizing the customer experience, which can be a game changer for ecommerce. Powered by AI algorithms and customer data, these recommendations help deliver tailored experiences, increase customer satisfaction, and drive revenue growth.
In our article, you will learn more about this technology: how it works in the Yespo omnichannel CDP and what you need to know to use it effectively. Moreover, we will look at several case studies demonstrating the results of personalized recommendations.
Essentials of Personalized Product Recommendations
What is a recommendation system
A recommendation system is
a type of software that analyzes a user's past behavior, preferences, and interactions with products or services and then generates personalized recommendations based on this data. The goal of a recommendation system is to help users find products or services that they are likely to be interested in but might not have discovered on their own.
Let's take a quick look at the process of creating personalized product recommendations:
- Data collection – a product recommendation system relies primarily on a large amount of complex information. This involves collecting data on user interaction with the website, application, and communication channels. The system takes into account customers personal information, as well as attribute data related to products.
- Data unification – information gathered from various sources may come in different formats — structured, semistructured, or unstructured. It is essential to standardize, deduplicate, and unify this data. After processing, all data points are accumulated into a single user profile for further use in segmentation, analysis, recommendation generation, etc.
- Data processing – understanding customer preferences, behavioral patterns, and connections between items requires solid data processing capabilities and analytical methods such as machine learning and deep learning algorithms.
- Generation of product recommendations by AI algorithms – AI algorithms generate offers by analyzing all available information about a customer, statistics about consumers with similar behavior and preferences, product data, and more.
- Omnichannel usage – the generated recommendations are displayed in real time on the website pages, within the app, and across direct channels chosen by the marketer (email, push notifications, app inbox, rich messages, etc.).
An omnichannel customer data platform is best suited for all of the above processes. It provides functionality for tracking, collecting and storing information about how consumers interact with the brand at different points. Data is then unified and can be used for purposes like segmentation, recommendation, analytics, etc. The combination of these CDP capabilities helps companies communicate with each customer most effectively. Read more about all CDP opportunities.
How product recommendations help solve ecommerce main issues
Companies experiencing a lack of customer personalization often face obstacles that can limit their growth and profitability. However, the strategic implementation of product recommendations can help solve some of these problems.
- Improving Customer Retention Rate
It is one of the biggest challenges companies face. If customers can't find products that meet their needs and desires or if they feel disconnected from the brand, customer loyalty declines. Personalized recommendations act as a compass, guiding users to individual choices. Utilizing data about past purchases, browsing history, and customer preferences, companies can create recommendations that generate genuine interest and foster a sense of brand connection. Yespo's developers can customize the neural network to predict the products that customers are likely to buy with up to 60% accuracy.
- Reducing Cart Abandonment
Abandoned shopping carts are a major headache for businesses. Various factors, such as unexpected additional costs or customer uncertainty about the product selection, can lead to this unpleasant situation. Personalized recommendations help by offering a range of similar products at different price points. Suggesting options that match the customer's preferences eliminates doubts and encourages the customer to complete the purchase.
- Increasing Average Order Value (AOV)
Companies struggling with low average order values often face a revenue problem. Customers who spend the minimum amount per transaction reduce profits. Personalized recommendations are key to improving AOV. In fact, 25% of respondents who participated in the study found that ecommerce personalization increased AOV. By analyzing a customer's purchase history, preferences, and additional products, companies can offer relevant options to increase upsells and cross-sells.
- Enhancing the Margin of Main Product Group
If the company's main product group has low profitability, personalized product recommendations can significantly affect the overall profitability of the business. They are aimed at cross-selling, promoting high-margin accessories or complementary products that enrich the customer experience while increasing profits.
Marketers can manage the conditions for adding products to recommendation blocks without involving developers. In addition, the Yespo team will develop custom algorithms if they are needed. This way, the neural network learns to recognize unique patterns that best suit a company's needs.
Product Recommendations in Detail
What data is used in a recommendation system
A recommendation system uses all available data to generate personalized offers, including the following.
Interaction data. It’s all about how users interact with products or services, such as clicks, likes, and purchases.
Important
Behavioral data is crucial for generating recommendations. Yespo CDP is able to collect this information itself from websites, apps, direct channels, and offline and update it in real time.
To collect this data from online stores and campaigns, we ask customers to install Yespo's web tracking script and set up main tracking events, such as:
- PageView;
- ProductPage;
- CategoryPage;
- StatusCart;
- PurchasedItems.
Use the Yespo SDK to track events in mobile apps. After the integration of our API methods library, all important behavioral data in the app is collected and sent to the CDP automatically.
User data: demographic information, location, personal preferences, size, etc. This data can be used to provide highly accurate recommendations. For example, algorithms can recommend only products that are available in the user's location.
Item data: attributes of goods or services, such as price, category, and brand. Algorithms get this data from the product feed.
You can additionally enrich the data used to generate recommendations with information from external sources, such as Google BigQuery, Google Firebase, PostgreSQL, and CRM systems.
The more information a company collects about its customers, the more accurate product recommendations can be generated for each individual. It is also important that the data should be transmitted in real time, so that algorithms can show offers on the website and in the app that match the interests of the visitor at any time.
How deep learning is used in recommender systems
You can create personalized offers using a variety of data analysis and clustering tools. However, the most effective suggestions are built using deep learning AI. Big data is needed to enable AI algorithms to generate recommendations that are relevant to each consumer. In turn, deep learning is used to improve their accuracy and performance. One of the advantages of using deep learning in recommender systems is that it can model non-linear relationships between users and items. For example, a deep neural network can learn to recognize complex patterns in user behavior and make personalized recommendations based on those patterns.
Here is a detailed explanation of how deep learning is used in recommender systems:
- Data Preprocessing: The initial step in constructing a deep learning-based recommendation system involves data preprocessing. This typically encompasses cleansing and transforming raw data into a format suitable for utilization by the deep learning model. For example, data could be converted into a user-item matrix, where each row corresponds to a user, and each column corresponds to an item.
- Data Representation: The next step is to represent the data in a way that the deep learning model can use. One popular approach is to represent users and items as vectors in a high-dimensional space. These vectors can be learned using techniques like matrix factorization or by training a deep neural network.
- Neural Network Architecture: Once the data has been preprocessed and represented, the next step is to design the neural network's architecture. You can use many different architectures, but one popular approach is a multi-layer feedforward neural network with one or more hidden layers. Typically, the user-item vector serves as the neural network's input, producing an output score representing the predicted items.
- Training the Model: The next step is to train the deep learning model. This involves feeding the training data (i.e., user-item vectors and corresponding ratings) into the neural network and adjusting the weights of the network to minimize the prediction error. This process is typically done using stochastic gradient descent or a similar optimization algorithm.
- Making Predictions: Once the deep learning model has undergone training, it can generate predictions for new user-item pairs. This involves feeding the user-item vector into the network and computing the output score. The items with the highest scores are then recommended to the user.
Each neural network has its own task, depending on the business goal. For example, algorithms can learn to match products that are commonly purchased together or predict the customer's next purchase. As a result, the dataset used to train the neural network and its architecture is tailored differently depending on the task.
Important
During several years of testing, Yespo's specialists have developed 180 AI algorithms for generating recommendations suitable for companies of various types and orientations. They are implemented in the system and are available for use.
However, the network is always learning and training on a specific company's data in order to make accurate recommendations.
Once trained, the neural network is deployed in the working environment (website, application). Now it can be used in several recommendation blocks simultaneously, where products are selected according to different logic. For example, to show only discounted products, or items of a certain brand, or in a certain price range, etc. Importantly, the model does not need to be retrained for such different purposes, you only need to adjust the recommendation filters.
Types of recommendation system algorithms
Artificial intelligence creates personalized recommendations by analyzing many different parameters, both customer behavioral data and product information. Let's take a closer look at the types of product recommendation engines Yespo has in place.
1. Content-based recommendation systems suggest products that are similar to those the user has interacted with or purchased based on item attributes. This algorithm operates by using product attributes (e.g., descriptions, metadata, etc.) to recommend items that are similar to the user’s prior interests. This method is useful for recommending niche items or in situations where there is limited user interaction data. For example, in the early stages of a relationship, before enough data has been collected for more sophisticated algorithms.
2. Collaborative filtering recommendation systems suggest items based on the behavior of users who share similar tastes or on the likeness between products. The idea behind collaborative filtering is simple: if you and someone else have liked similar things in the past, you're likely to enjoy similar things in the future. There are two main types of collaborative filtering:
- User-based — this method compares your behavior to other like-minded users and suggests products they've enjoyed.
- Item-based — instead of users, it looks at product similarity. It suggests items similar to ones you've already shown interest in or bought.
3. Hybrid recommendation systems combine different methods to produce more accurate and personalized suggestions. For example, a hybrid system might use collaborative filtering to offer items based on user behavior and content-based filtering to suggest items based on item attributes.
4. Personalized recommendations offer tailored suggestions to individual users based on their specific interests, preferences, and behaviors. These recommendations are customized to each user's unique characteristics and tend to deliver higher customer engagement and satisfaction compared to non-personalized alternatives.
The process of generating personalized recommendations typically involves the collection and analysis of data related to each user's activities on websites and mobile apps, including:
- browsing history;
- search queries;
- purchase history;
- favorites list, and other relevant data points.
Then, the system uses this data to create a user profile, which is used to predict the products or services that are most likely to capture the user's interest. We use deep learning techniques to create these personalized recommendations.
5. Session-based personalized recommendations are a type of recommendation system that provides personalized recommendations based on a user's recent interactions within a single browsing session. The algorithms process this sequence of events and learn to predict the user's next action, which can be used to generate more contextually relevant personalized recommendations in real time.
Recommendation blocks types
Recommendation blocks are sections on a website, app, and emails where product offerings are displayed to users. They are strategically designed to enhance the likelihood of a user making a purchase by presenting items aligned with their potential interests. Here are some common types of recommendation blocks:
1. Similar (or related) products: This type of recommendation shows other items that are similar to the one the user is currently viewing. For example, if a visitor is viewing an out-of-stock product, you can offer them to look at similar items.
2. Frequently bought together: This type of recommendation suggests products that are often purchased together with the item the user is viewing. For example, if someone is looking for Halloween decorations, the products often purchased together may include a wreath in matching colors, candles, decorated textiles, etc.
3. Popular / Trending products or bestsellers: This type of recommendation showcases the most popular products on the website. This can help users discover items that are trending or in high demand.
4. Recently viewed items: This type of recommendation shows products that the user has recently viewed. This can be helpful in case the user wants to return to a product they have previously seen.
5. Personalized recommendations: This type of recommendation uses data on the user's browsing and purchase history to suggest products that the user is likely to be interested in.
6. New arrivals or discounts: This recommendation type introduces recently added products or discounted items, assisting users in staying current with the latest offerings and securing the best deals.
Application of product recommendations in different channels
Using product recommendations, an ecommerce company can engage with customers across various touchpoints, delivering omnichannel personalization:
- On a website. Seamlessly integrated into a website's interface, recommendations guide users through a curated selection of products based on browsing behavior, preferences, and historical interactions. They enhance user experience and boost conversions.
- In a mobile app, personalized recommendations provide users with a customized shopping journey. By analyzing in-app activities, these suggestions lead to increased user engagement and higher retention rates.
- Offline stores, using product recommendations, empower sellers with data-driven insights to offer personalized suggestions to customers, increasing upsell and cross-sell opportunities. This technology likewise helps customer service operators.
- In direct channels, personalized offers encourage subscribers to visit the website or app and drive cross-selling and upselling. For this purpose, ecommerce uses direct channels:
- email;
- web and mobile push notifications;
- app inbox;
- rich messages.
In emails, content personalization based on user preferences increases the relevance of promotional messages and fosters stronger customer relationships.
Push notifications, enriched with personalized suggestions, create real-time engagement opportunities. Users are enticed with products that match their interests, encouraging immediate action and building brand loyalty.
With Yespo CDP solutions, you can include up to 10 images in a mobile push, showcasing a single product from different angles or featuring multiple items.
Our statistics show that you can increase the open rate up to 2-4 times by using several images in a push notification.
App inbox is a notification center in the personal account of a registered user of your website or app. In this channel, you can recommend a single product or guide a customer to a page with a personalized assortment. Similarly, you can include product recommendations in rich messages, presenting the best offer or inviting a person to explore a personalized selection.
Increase revenue from marketing channels using product recommendations
Commonly used page types and related recommendation blocks
Recommendation blocks can be used on various high-traffic pages in an ecommerce website to improve the user experience and increase sales. Here are some of the page types and their suited recommendation blocks:
- Product Page displays detailed information about a specific item. For this type, it is recommended to use ‘Similar Products’ and ‘Frequently Bought Together’ blocks. Such blocks can assist users in discovering products they might not have come across otherwise, thereby increasing the likelihood of finding items they wish to purchase.
- Category Page shows a list of products within a particular category. For this page, it is recommended to use ‘Bestsellers’ and ‘Trending Products’ blocks. They utilize popularity and trends to recommend products that are in demand in the category.
- Home Page is the first page a customer sees when visiting an ecommerce website. For this page, it is recommended to use ‘Recommended for You’ or ‘Personalized Recommendations’ blocks. These use data about the customer's browsing and purchasing behavior to offer products that are most likely to interest them.
- Cart Page or Checkout shows the items that a customer has added to their cart. For this page, it is recommended to use ‘Frequently Bought Together’ and ‘Upsell’ blocks. These blocks display products that are often purchased together with the items in the shopping cart or higher-priced goods that are similar to the items in the cart.
Use Cases of Personalized Product Recommendations
Many of Yespo's clients, including major retailers, have been using personalized recommendations across multiple customer touchpoints for years. Learn more about the problems and challenges companies solve and the successes they have achieved with the product recommendations tool.
Pampik online store achieved a 1734% ROI increase
Pampik is a Ukrainian online store specializing in children's and maternity products. The monthly traffic of the site is 245 thousand visitors. In 13 years of operation, it has fulfilled more than 100 million orders.
The goal was to boost sales through cross-selling, upselling, and elevating the user experience for site visitors.
Solution. The decision was to implement ecommerce product recommendations across the site. We have defined the placement of the recommendation blocks as follows:
- Home page — showcasing popular products.
- Product pages — featuring 'Bought with this product' and 'Similar products'.
- Out-of-stock product pages — displaying similar products that were purchased alongside the unavailable item.
- Shopping cart — showcasing products commonly purchased with the selected item.
- 404 page — offering personalized 'Popular for you' recommendations.
- Thank you page — providing personalized 'Popular for you' recommendations.
Results. Over the course of a year, we achieved the following milestones:
- The ROI for product recommendations increased from 341% (at the beginning of its use, during the low season) to 1734% (culminating in the high season of the first year).
- 47.24% of the total revenue generated by recommendations comes from the blocks displayed on the home page.
- 24.8% of the total revenue generated by recommendations comes from the ‘Buy with this product’ block.
Retailer Antoshka boosts monthly revenue by 20% via recommendations in email
It is one of the leading retailers of children's products in Ukraine. The retail network has more than 40 offline stores in the country. The website has 700 thousand visitors per month.
The goal was to amplify sales through automated and personalized email campaigns. To achieve this, our strategy involved the implementation of behavioral triggers based on consumers' interactions on the website.
Solution. Collaborating closely with the client's marketing team, Yespo developers have implemented a number of personalized recommendation algorithms covering the following categories:
- 'You may also like'
- ‘Discounted items’
- ‘Items within the same category’
- Cross-selling
- Out-of-stock recommendations
Email recommendations are tailored to the age and gender of the subscriber's child, with each trigger containing between 3 to 6 suggested products. Furthermore, recommendation blocks are also displayed on the website. They are strategically positioned on the homepage ('Great Deals') and product cards ('Similar Items').
Results. The result of our efforts was as follows:
- The monthly revenue from the email channel increased by 20% due to behavioral triggers with recommendations.
- In comparison to mass emails, personalized campaigns have more than x2 higher Open Rate (OR) and Click-Through Open Rate (CTOR), alongside a 70% increase in transaction rates.
- The recommendation blocks embedded on the website prolong users' time spent within the online store and increased the number of viewed product pages.
Retailer Dnipro-M achieves a 23.5% ROI increase
It is a Ukrainian developer and seller of construction tools with a network of its own stores in five European countries. Two million users visit its online store each month.
To enhance customer loyalty, the company employs product recommendations in email campaigns and on its website. The primary challenge was to leverage these recommendations to drive sales growth.
Solution. We added recommendation blocks into the ‘Abandoned Cart’ and ‘Abandoned View’ omnichannel triggers. On the Dnipro-M website, product recommendations are presented as follows:
- Product cards feature similar items.
- The home page showcases bestsellers, popular products, and recently viewed items.
To further enhance the user experience, we added more personalized algorithms. This involved adding promotional bestsellers and personalized selections to the home page. Additionally, a ‘Buy with this product’ section featuring complementary items was introduced to the product page.
Results. During September-October 2022, omnichannel triggers as a percentage of total orders increased:
- Abandoned cart — 19 times;
- Abandoned views — 10 times.
The implementation of enhanced product recommendations on the website led to notable sales improvements:
- The share of total orders attributed to product recommendations on the main page increased by 2.4 times.
- On the product card, this share increased by 2.2 times.
Overall, ROI in product recommendations increased by 23.5%.
Omnichannel retailer Foxtrot reaches 16% growth in sales of accessories
Foxtrot is one of the biggest consumer electronics and housewares retailers in Ukraine. The network consists of 119 physical stores across the country. The retailer has a mobile app and an online store. 5 million users visit the site every month.
The company's challenge was to increase the margin of its main category of electronic products. To overcome this obstacle, the marketing strategy focused on increasing sales of related products and accessories. The key role of product recommendations on the website was to encourage customers to take targeted actions and increase the number of items in the check.
Solution. Yespo's developers have trained a neural network to predict customers' most likely next purchases. The work was done in close collaboration with data scientists. They ensured that only relevant offers were added to the recommendations. Additionally, this work required the involvement of client representatives, particularly for complex algorithmic setups. This collaborative effort was manifested in the 'Cheaper together' product selection block, where AI estimated the probability of purchasing items together and then created complete sets. The client's team checked the category relevance, cut off unnecessary products, and calculated the cost of the sets.
Blocks of personalized recommendations were strategically placed throughout the online store:
- Home page — 'Recommended accessories for your last purchase,' 'Special for You.'
- Category pages — 'You’ll be interested in.'
- Product cards — 'People also buy this product,' 'Selected based on your preferences,' 'Customers who viewed this product also consider.'
- Shopping cart — 'You may also need this product.'
Going beyond the personalization in ecommerce, Foxtrot has strengthened its omnichannel customer communication strategy by rolling out recommendations at bricks-and-mortar locations.
Results. The integration of product recommendations helped to achieve these outcomes:
- Accessory and related product sales increased by 16%.
- Page views and site engagement witnessed a 10% increase.
- The website conversion rate increased by 5%.
Retailer Comfy case: personalized blocks drive 49% of orders in promo emails
It is one of the largest retailers of electronics and home appliances in Ukraine. Comfy operates through a network of 94 stores, a mobile app, and an online store. The website has 8 million monthly visitors and consistently ranks among the top five online players in the Ukrainian market.
The company aimed to enhance order volumes through email personalization.
Solution. The Yespo team implemented personalized product recommendation blocks into both triggered and mass email campaigns. Our developers set up algorithms with customized filters to meet the business goals of the email campaigns. These filters include:
- products consistently displayed in recommendation blocks;
- priority products for increased visibility;
- exclusion criteria for certain product attributes, and more.
Recommendations were added into 9 types of triggers, such as price reductions for viewed products, wishlist recommendations, and post-sale notifications. In mass email campaigns, recommendation blocks enriched the 'Product of the day' and 'Day/Night Sale' newsletters.
Results. In the first two months since we implemented recommendations, we achived the following results:
- Personalized blocks drive 49% of total orders in promotional emails.
- Recommendation blocks in mass emails achieved a CTR of 41%.
- Personalized recommendations in triggers bring 20% of orders.
- The share of triggers with recommendations in the total revenue of the email channel is 13%.
Conclusion
Personalization in marketing is crucial, especially in the retail industry. By leveraging the power of AI algorithms and valuable customer data, product recommendations provide an individualized shopping experience, improve customer satisfaction, and increase revenue.
Data is the foundation of recommender systems, and we explored what kind of information is needed to deliver a personalized customer experience. The ability to utilize big data is necessary for AI algorithms to provide recommendations tailored to individual consumers. In addition, deep learning improves the accuracy and performance of these algorithms. There are different algorithms for recommender systems, each designed to solve specific tasks and business goals. We explored the types of recommendation blocks and their role in shaping the user experience and illustrated how they can be used strategically on different web pages to increase engagement and sales.
Our clients' case studies demonstrate the ability of recommender systems to solve critical retail problems. Thanks to the personalization engine from Yespo, you can overcome the issue of abandoned carts and abandoned views, as Dnipro-M did. Improve customer engagement on the website, like Antoshka, and increase the profitability of the email channel, like Comfy. Generate revenue through upselling and cross-sales, like Pampik or increase the average order value and improve margins in key product categories, like Foxtrot. Finally, increase revenues in general, as our clients have done.
If you're interested in learning more about how your business can achieve the same or better results with our help, contact us at info@yespo.io. Or, schedule a demo right now, where Yespo specialists will demonstrate the CDP's capabilities in action and answer all questions about your personalization strategy improvements.