22 September 2023
1182
13 min
5.00
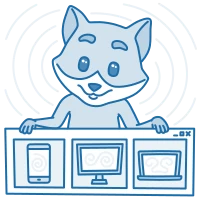
Foxtrot Case Study: +16% Accessories Sale with the Same Traffic
The success of the business largely depends on the quality of communication with customers. To date, there are many tools that allow you to keep track of any changes in user behavior. But what about predicting future purchases and creating customized product offerings based on the data?
In this case study, we will explain how one of the top Ukrainian electronic retailers increased accessories sales by 16% using personalized recommendations.
About the Project
Foxtrot is one of the largest retail stores of electronic and household appliances in Ukraine. The trading network is part of Euronics, the largest association for the purchase of electronics and household appliances, which covers 37 countries, including most European countries, Kazakhstan, the UAE, Egypt, and others.
Foxtrot is represented by 164 stores in 90 cities of Ukraine and online (average site traffic for 6 months is 5.58 million users).
The FoxFan loyalty program has more than 11.5 million customers, and 92% of purchases are made with a network card. The secret to the brand’s success is personalization: all loyalty program participants receive relevant offers, bonuses, as well as the chance to buy a product at a special price (depending on the stage of the funnel and a possible discount on the item of interest).
Foxtrot and Yespo have been cooperating for more than 7 years, during which time we have jointly:
- implemented email campaigns;
- connected omnichannel communication using pop-ups, SMS, Viber, and push notifications;
- configured 25 omnichannel workflows and 80 triggers.
The retailer continues to optimize marketing processes and improve communication with the target audience.
Task
In the electronics business, the margins of the main product category are low. Therefore, the main task for Foxtrot was to increase sales of additional and related items.
That’s why they decided to implement product recommendations on the website to increase:
- number of conversions and targeted actions on the site;
- number of purchased items per order;
- sales of accessories and related products from cross categories.
The Solution
“Despite having an IT department that can implement any functionality, we decided to cooperate with Yespo. In some cases, it is not reasonable to spend time and effort on developers if there is a ready-made solution on the market and you can start using it immediately. In addition, Yespo is continuously improving its product, and we can use the most advanced tools.” — Evgeniy Krechetovich, Head of CRM at Foxtrot
Product recommendations in Yespo are created thanks to the synergy of artificial intelligence and data science experts. A neural network can predict what products your consumers will buy with 60% accuracy. To ensure that recommendations are relevant, this process is controlled by an analyst who sets up and trains the system further.
There is no universal algorithm: some of the product recommendation approaches are more effective for an electronics retailer, and others are more effective for an online clothing store. Therefore, the data scientist, together with the project manager and the customer, determines the categories that require special attention based on the needs of a particular company.
Discover product recommendation approaches that suit your business!
How Does It Work
Recommendations are generated by a neural network based on:
- behavioral history of a large sample of buyers;
- information about products and their characteristics.
In Yespo, you can configure the following types of algorithms:
Algorithms |
Pages | Data sources |
General algorithms Show only those products customers buy most frequently |
For any pages |
Use general website data without personalization about bestsellers, and the most popular products |
Product-based algorithms Use data about the products |
For pages showing a specific product or category |
Personalized recommendations from category are based on views. Users who bought this item also viewed are based on clicks. Customers who added to the cart also added – name, price, category, and description are analyzed. Bought with this product are based on the purchase data. Similar products are based on the category, description, name and price of the product. |
Customer data-based algorithms Create unique recommendations for every user |
For any pages |
Similar products are based on the category, description, name, and price of the product. Personalized recommendations is a complex algorithm that analyzes customer behavior in categories and on product cards. |
Foxtrot has personal recommendations in the following blocks:
- main page: “Recommended accessories for your latest purchase”, “Especially for you”;
- category pages: “You might be interested”;
- product cards: “Bought with this product”, “Selected based on your requests”, “Customers who viewed this product are also interested”;
- cart: “For this product you may need”
To form them, the neural network takes into account:
- history of user behavior on the site;
- products cost;
- product category and related categories;
- additional conditions from the client.
Product Recommendation Specifics on the Foxtrot Website
The store’s assortment includes more than 10,000 accessories – only artificial intelligence can handle such a volume of data. But since the data structure of different products is not always the same, this can cause difficulties for AI and reduce the accuracy of recommendations. Therefore, data specialists should explain to the system how to recommend relevant products. Let’s look at examples of several categories on the Foxtrot website:
- TV mounts – to choose the right wall mount, you need to consider the weight of the TV and the standard, such as VESA. Sometimes the data on product cards may be incomplete (in the specifications of some TVs the weight is indicated with a stand, while in others – without it). In such cases, an ML algorithm is used to clean this data and select stands for all TVs.
The data scientist further trains the algorithm and indicates weight as a key characteristic for both the TV and the stand. Once this information is stored, the AI recommends the correct mounts.
Thanks to this adjustment of the algorithm, sales of TV mounts increased by 6%.
- TV tuners and set-top boxes – TVs may or may not be equipped with a TV tuner. This characteristic influences the formation of related positions groups. So, for TVs without this function, tuners can be recommended since they are usually viewed and purchased in one order. In the case of media players, the recommendations work similarly: they are relevant for those models that do not have Smart TV.
- Accessories for mobile phones. Their specificity is that they must clearly correspond to a specific phone model. But since there are a lot of offers in this category (cases, USB cables, memory cards, holders, etc.), there is a possibility of error. To exclude it, a specialist checks the algorithm’s recommendations and removes incompatible ones. For the AI to select the correct related products, the brand, model name, and accessory must match.
In April 2021 alone, recommendations brought +16% to accessory sales.
Create personalized product blocks on your website!
- Large appliances. As a rule, buyers choose household appliances of the same color and brand. The right recommended products for household appliances can generate significant income since there is a high average check compared to other categories. To create relevant recommendation blocks, a data specialist indicates to the neural network that the brand is a key characteristic. The fewer other brands included in the recommendations for a specific product, the higher the sales of related products will be.
To create the best recommendations, it is necessary to involve the efforts of the neural network, the project team and the business itself at the same time. For example, the creation of such a complex block as “Cheaper together” proceeds like this: AI calculates the probability of purchase and creates sets → on the client side, the category compliance is checked, irrelevant ones are excluded + the cost of the set (with margin and discount in mind) is calculated.
At the moment, using automation for Foxtrot, more than 500,000 sets have been generated in all product categories.
In a similar way, the block “Bought with this product” is created. The neural network analyzes information on previous orders → identifies non-obvious patterns (for example, one purchase periodically contains an iron and a set of glasses, a hammer drill and a coffee maker, a large TV and a heater, etc.) → builds sets → recommendations are displayed in product cards.
Every day new suppliers are added to the site, the range of thousands of products is updated, so the creation of such kits becomes possible only with the help of artificial intelligence.
If the algorithm cannot select relevant recommendations (for example, due to the lack of necessary characteristics of the products), then no recommendation is given on the Foxtrot website. Instead, the user is asked to independently explore products from a general category. The retailer and Yespo chose this strategy because incorrect offers could negatively affect audience loyalty.
Offline Product Recommendations
Foxtrot complemented its omnichannel approach to communication with customers with offline recommendations.
Blocks with kits are displayed at workplaces for call center operators and retail salespeople. This allows you to reduce the burden on staff and improve the quality of service. Now consultants do not need to memorize the entire assortment, which amounts to more than 80 thousand products, in order to quickly and accurately recommend relevant items to the buyer.
In addition, customer cards are enriched with information about offline views. To do this, the consultant takes the visitor’s phone number → enters the viewed products as an order → then the trigger chain is launched with the abandoned view.
Foxtrot encourage visitors to leave their phone number offline in the next ways:
- saving customers’ time – they can follow the links in the email and discover the characteristics instead of searching for items on the site (when shopping for electronics, it takes a longer time to decide on the purchase and customers get back to the product description more than once);
- the opportunity to get a special price for a product;
- convenience and no necessity to carry plastic cards.
Personalized recommendations for offline customers!
The Results
Effectiveness of recommendations for Foxtrot:
- +10% increase in viewing depth and engagement on the site;
- +5% conversion growth;
- +16% accessories sale growth.
“Product recommendations are a functionality that allows Foxtrot to offer customers the products they want to buy. We like how recommendations work and the results they bring, and together with Yespo, we are constantly improving them. The realization of all ideas occurs quickly and smoothly; there is no need to set deadlines. The team describes what they want to see, and specialists find solutions and help with implementation. At Yespo, we can always get high-quality expertise and help with settings.” — Evgeniy Krechetovich, Head of CRM at Foxtrot
Conclusion
Not only retail giants like Foxtrot can afford to connect product recommendations. Over many years of testing and working with different businesses, the Yespo team has identified the best algorithms and integrated them into our system. Any marketer can set up recommendations on a website independently without the help of third-party programmers. The results of personalized blocks are available immediately after they appear on the site, which will allow you to determine the most profitable recommendations. If you have any questions, fill out the form, and together, we will find the best solutions for your business!