31 January 2025
577
34 min
5.00
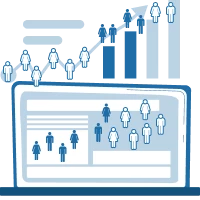
Predictive Segmentation 102: Technology and Metrics
The discipline of marketing has come a long way since its modest beginnings millennia ago. And so improved our tools. From clay tablets and paper notebooks to software spreadsheets and state-of-the-art artificial intelligence systems. We’re always striving to get better results and looking for new ways to achieve them. At the same time, we don’t want to do tedious work, and it’s understandable—with so much on our plates, we just can’t afford to do everything manually.
This is why the marketing automation field sees explosive growth. We create content and copy with the aid of AI, we generate personalized product recommendations, and we automate routine tasks and workflows. All to get better results while spending less time.
Customer segmentation is a part of the equation that was difficult to automate. At least until recently. However, with the emergence of predictive segmentation (which we covered earlier), we can group customers on autopilot—while achieving superior results.
In this article, we’re going to explore its underlying technology so you know everything you need to get better outcomes. And we’ll try to make it as accessible as possible, so those without a degree in advanced statistics won’t fry their brain cells. You have other things to occupy your mental bandwidth, right?
Basics of predictive segmentation vs. descriptive methods
Segmentation is an important technique in marketing. Its purpose is to separate a customer base into distinct groups based on shared characteristics. Traditionally, it’s been done manually based on business objectives. For example, a certain product can be advertised exclusively to women. Or a promotion being shown only for users on mobile devices.
These methods can also be referred to as descriptive, as they focus on grouping existing customer data (i.e., “describing” it).
We’ve covered some of the most common approaches in our previous article. But here’s a quick recap of these methods:
Demographic segmentation
This fundamental approach divides customers based on shared traits of customers, like age, gender, income, and education level. For example, a luxury brand might target high-income professionals in their 40s, while a fast-fashion retailer focuses on younger customers with moderate incomes.
Companies can create highly specific combinations of these factors, such as "married women aged 25-34 with graduate degrees," to target precisely their marketing efforts and product development.
Geographic segmentation
Location-based targeting considers not just physical location but the entire context of where customers live and work. This includes urban versus rural settings, climate conditions, population density, and cultural preferences unique to specific regions.
Modern approaches like geofencing made it easy for businesses to reach customers at specific locations in real time.
A retail chain might adjust its product mix based on local weather patterns, or a restaurant could modify its menu to accommodate regional taste preferences. This method is particularly valuable for businesses expanding into new markets or optimizing their distribution networks.
Psychographic segmentation
This deeper analysis examines the psychological aspects of consumer behavior, including lifestyle choices, personal values, interests, and attitudes. It answers questions about why customers make certain choices by grouping them into categories like "health enthusiasts," "tech early adopters," or "environmentally conscious consumers."
Companies use this information to craft marketing messages that resonate with their target audience's core values and aspirations, creating stronger emotional connections with their brand.
Behavioral segmentation
By analyzing how customers interact with products or services, businesses can group them based on their actions rather than their characteristics. This includes purchase frequency, brand loyalty, usage rate, and response to marketing initiatives.
For instance, a software company might separate power users from occasional users, or a retail store might identify bargain hunters versus premium shoppers. This segmentation helps develop targeted retention strategies and personalized campaigns.
Value-based segmentation
This method focuses on the economic relationship between customers and the business, considering factors like customer lifetime value, purchase frequency, and average order value. Companies use this approach to identify their most profitable customer segments and understand what makes them valuable.
This information guides strategic decisions about resource allocation, helping businesses invest more in acquiring and retaining high-value customers.
RFM segmentation
This powerful approach combines three key metrics to evaluate and segment customers:
- Recency—how recently they made a purchase.
- Frequency—how often they buy.
- Monetary value—how much they spend.
For example, a customer who bought something last week, shops monthly, and spends significant amounts would be considered highly valuable. RFM helps businesses identify their best customers, spot those at risk of churning, and create targeted marketing campaigns for each segment.
It's particularly effective for retail and ecommerce businesses looking to optimize their customer engagement and retention strategies.
Which segmentation method is right for my business?
How predictive segmentation works
While descriptive segmentation relies on manually defined rules and clear-cut criteria ("customers who spent over $100 last month" or "women aged 25-34"), predictive segmentation uses an entirely different approach.
Instead of using fixed rules, it analyzes patterns in historical customer data to forecast future behaviors. For instance, rather than just looking at past purchases, it can identify subtle trends that indicate a customer is likely to buy again soon.
The power of this approach lies in its ability to process vast amounts of interconnected data points. When a marketer creates segments, they typically focus on 3-4 key variables at most—it's simply too complex to consider more.
Machine learning algorithms, however, can simultaneously analyze hundreds of variables: not just obvious ones like purchase history and demographics, but also minor changes in browsing behavior, response to previous campaigns, seasonal trends, and even the time between website visits.
These algorithms don't just look at individual variables in isolation. They identify complex relationships between different factors. For example, a predictive model might discover that customers who browse your site on weekday evenings, regularly open your emails, and have made at least two purchases in different categories are highly likely to respond to your next promotion. These patterns would be nearly impossible to spot through manual analysis and are unique for each business.
Past vs. future: A fundamental difference
The most important distinction between descriptive and predictive segmentation lies in their relationship with time. Descriptive segmentation is inherently backward-looking—it can only tell you about what customers have already done or who they currently are.
When marketers create rules like "customers who purchased in the last 30 days" or "visitors who abandoned their cart," they're essentially using history to guess future behavior.
Predictive segmentation, on the other hand, directly answers the question that really matters to businesses: "What will this customer do next?"
Instead of assuming past behavior will continue, it actively forecasts future actions. Rather than creating a segment of "customers who bought frequently in the past," it identifies "customers likely to make a purchase in the next two weeks"—even if some of those customers don't fit the traditional profile of a frequent buyer.
This shift from descriptive to predictive analysis fundamentally changes how businesses can approach their marketing. Instead of reacting to past behaviors, they can proactively engage with customers based on their likely future actions.
Nuts and bolts of predictive segmentation
To understand how predictive segmentation works, let's look under the hood. While you don't need to be a data scientist to use this technology, knowing the basics will help you make better decisions about when and how to use it in your marketing.
Ready? Let’s go!
The data pipeline
Predictive segmentation is powered by data. The more data you have, the better. And it’s quality and completeness directly affect the accuracy of predictions.
What data you need
Predictive models deliver the best results when they can consider the complete customer picture. While each business is unique, there are several key types of data that drive effective predictions:
Behavioral data
- Website and app interactions: Pages viewed, time spent, features used
- Campaign engagement: Email opens, clicks, responses to different offers
- Content preferences: What articles they read, videos they watch, products they browse
- Search patterns: What they're looking for on your site
Transactional data
- Purchase history: What they buy and when
- Order values: How much they typically spend
- Product preferences: Categories and specific items they favor
- Payment methods: How they prefer to pay
- Subscription status: If they're on a recurring plan
Customer attributes
- Basic demographics: Age, location, gender
- Contact information: How they prefer to be reached
- Account details: How long they've been a customer
- Custom fields: Any specific data points important for your business
- Loyalty program status: If you have one
The best part? You don't need all of this data to get started—predictions can work with whatever information you have available. As you collect more data over time, the results become more accurate and nuanced.
Data sources and flow
If you’re using at least one software solution to automate your marketing, you’re already collecting valuable customer data. However, before this data is passed to your predictive segmentation system, you have to ensure it’s all properly collected and stored.
Usually, it’s better to have one central location that unifies data from all of your existing data sources. Customer Data Platforms (CDPs) are perfect for this.
CDP acts as a hub that collects all your data in one place and uses it to create predictions (unless you rely on a third-party solution). It allows you to:
- Connect data from multiple sources into a single customer view
- Keep customer profiles updated in real-time
- Ensure data consistency across different systems
- Make data accessible for predictions when needed
To enrich your CDP data, you‘d want to connect other data sources, such as:
- CRM systems: Customer information and interaction history
- Analytics platforms: Website and app behavior
- Marketing tools: Campaign engagement and responses
- Support systems: Customer service interactions
- Custom databases: Any other data you collect
Keep in mind that you don’t just collect data once—for the best results, you need a system to maintain a continuous flow of information, including:
- New purchases
- Customer behavior
- Campaign responses
This continuous flow allows your predictive segments to stay current and reflect your customers' most recent behavior and preferences. For example, if a customer starts showing signs of decreased engagement, they can be automatically moved to a different segment that might need special attention.
Collect all your marketing data in one place
Why clean data matters
Remember Justin Timberlake’s “What Goes Around... Comes Around?” With predictive segmentation, it isn’t much different. The quality of your input data directly affects the quality of predictions made by a system.
Common issues include:
- Duplicate customer records
- Missing or incorrect information
- Inconsistent formatting
- Outdated data
This is why most predictive systems include automatic data-cleaning steps. They standardize and verify the data before using it to make predictions.
Did you know?
Yespo CDP automatically cleans and prepares data needed for segmentation. If you performed all technical setup steps, such as installing a web-tracking script, you don’t need to do any additional data cleanup.
Model building
Once the data is ready, the system starts looking for patterns that can help predict future customers’ behavior. Think of it as connecting countless dots across your customer data to reveal a clear picture of what different actions mean for their future actions.
The system can use various types of models depending on what you want to predict. The most common type for customer behavior is classification—where the goal is to sort customers into binary groups based on what they're likely to do next (e.g., buyers vs non-buyers). Other models might look for natural groupings of similar customers (clustering) or try to predict specific values like future purchase amounts (regression).
For marketing purposes, classification models are particularly valuable because they answer practical questions like "Will this customer buy in the next 30 days?" or "Is this customer likely to churn?" They do this by learning from past examples of customers who did or didn't take these actions.
How the system learns
The system analyzes historical data to understand what happened in the past. Let's say you want to predict which customers will make a purchase in the next month. The system looks at two groups of customers from the past:
- Those who did make a purchase within 30 days
- Those who didn't make a purchase in that timeframe
For each group, it examines hundreds of different signals:
- How recently they visited your website
- Which pages they looked at
- Whether they opened your emails
- Their past purchase patterns
- How they interact with your brand
- And many other data points
Over time, the system begins to recognize which combinations of these signals most reliably predict future purchases. Some patterns might be obvious, like frequent website visitors being more likely to buy. Others might be subtle connections that would be impossible to spot manually—like customers who browse your help articles being more likely to make larger purchases.
The same process applies to any customer action you want to predict, whether it's churn, upgrades, or engagement with specific products. The system continuously learns from new data, refining its understanding of what different customer behaviors mean for future actions.
Converting patterns to predictions
This is where classification comes in. It puts customers into different groups based on how likely they are to take a specific action. For instance, the system might classify customers as:
- "Very likely to purchase" (80%+ probability)
- "Likely to purchase" (50-80% probability)
- "Unlikely to purchase" (20-50% probability)
- "Very unlikely to purchase" (less than 20% probability)
These probabilities are based on how closely a customer's current behavior matches patterns that led to purchases in the past. For example:
- A customer who visits your website three times in a week, opens all your emails, and looks at pricing pages might show an 85% probability of purchasing.
- Someone who hasn't opened your emails in two months and only visited once might show a 20% probability.
- A customer who recently viewed similar products multiple times but hasn't purchased might show a 60% probability.
The system updates these predictions continuously. A customer who was "unlikely to purchase" last week might move into the "might purchase" category after engaging with your latest email campaign. This dynamic nature means your segments are always current, reflecting the latest customer behavior.
With predictive models, you can run effective and profitable campaigns while spending less time tweaking and updating your segments manually. However, not every model produces equal results, that’s why we need a way to assess their performance and outputs. This is where evaluation metrics come into the picture.
Model evaluation
To better understand how to evaluate predictive models, imagine this scenario: You have a large haystack in front of you. See where it’s going? Yes, it’s about needles. Not one needle, but a lot of needles in that haystack that you need to find.
However, the needles aren’t all alike. Some of them are longer, some are thicker, and yet some are even curved! Not the easiest task, right?
The Confusion Matrix
Given the objective, you devise a special sieve, that filters your hay and finds needles in it. This is your predictive model, so to speak. Yet, given that needles are different and hay is not uniform either, sometimes it will leave needles in the hay, and sometimes hay will pass through as if it were a needle.
In predictive modeling, we have an instrument called the confusion matrix, which describes this situation. It’s a 2X2 grid that covers these scenarios.
Here’s what it means:
True positive (TP): The model correctly predicts the positive class. It’s like when your filter correctly pulls a needle.
False positive (FP): The model predicted an outcome that wasn’t true. It’s when your filter pulls a straw instead of an actual needle.
False negative (FN): The model didn’t predict a true outcome. Consider a situation when your filter leaves a needle in a haystack, instead of correctly filtering it through.
True negative (TN): The model correctly predicts a negative class. Your filter leaves a straw where it belongs—in the haystack.
Metrics used to evaluate predictive models
When working with models (just like our filter for needles), it’s important to have some sort of measure by which we can evaluate the effectiveness of our solution. To do that, we have various metrics. These metrics are different and are used for different scenarios. Let’s take a closer look at the most common ones and when you should use them.
Accuracy
When it comes to model evaluation, accuracy is the simplest and most straightforward metric in our toolkit. It’s calculated as the ratio of correct predictions to the total number of predictions.
It’s a simple and easy-to-understand formula good for initial evaluation and balanced datasets (i.e. you have roughly the same number of needles and straws in your haystack).
However, accuracy falls short for more complex scenarios and imbalanced sets. Let’s consider an example: you have 5 needles and 95 straws. The model can flag everything as a straw, and the accuracy will be 95%. While this seems like a good result, in reality, we didn’t get a single needle from our sieve.
Due to this, accuracy is not the most reliable metric. Still, it has its uses for balanced datasets and situations, when errors are not critical.
Pros of using accuracy
- Simple, easy to understand, and quick to compute
- Works well for balanced datasets
- Widely applicable to different types of classification models
Cons of using accuracy
- Misleading for imbalanced datasets, where it can mask poor performance on minority classes
- Doesn't distinguish between different types of errors (false positives vs. false negatives)
- Not suitable when the costs of different errors vary
When to use accuracy
Balanced datasets: Accuracy is most effective when both positive and negative cases appear in similar proportions.
Low-cost errors: When false positives and false negatives are equally important or have a low impact (e.g., classifying spam emails).
Initial model evaluation: It is often used as a baseline metric for quick model assessment before moving on to more detailed metrics like precision, recall, or F1 score.
Precision
Precision is one of the two most important metrics used for the model evaluation process (the other is recall). It measures the accuracy of positive predictions and is calculated as a ratio of all correct positive predictions to all cases flagged as positive.
In most cases, it’s a more accurate metric than accuracy (pun intended), and it’s especially relevant for cases where false positives are more detrimental than false negatives.
Let’s get back to our haystack example. If our model identified 100 items as needles, and in reality, 90 were the actual needles (true positive), and 10 were straws (false positive), we can say that it has precision at 90%.
However, what precision doesn’t tell us is how many needles were left behind in the haystack. Imagine there are 200 needles in total, but the model only identified 90 of them. This means the model missed 110 needles (false negatives), which is critical information if we care about finding as many needles as possible. Precision alone doesn’t capture this aspect.
Pros of using precision
- Focuses on minimizing false positives, which is crucial when they are costly (e.g., spam filters, fraud detection)
- Provides actionable insights by ensuring predicted positives are reliable
Cons of using precision
- Doesn't account for false negatives, which can be problematic when catching all positives is important (e.g., medical diagnosis)
- Can be misleading in heavily imbalanced datasets
When to use precision
Precision is ideal in cases where false positives are more problematic than false negatives. Some specific examples include:
Identifying fraudulent transactions in financial systems:
- False positives (flagging legitimate transactions as fraudulent) can cause customer dissatisfaction, so high precision ensures that only truly fraudulent cases are flagged.
Spam email detection:
- High precision ensures that legitimate emails are not mistakenly marked as spam, preserving user trust.
Medical testing for rare diseases:
- In some scenarios (e.g., a highly invasive or costly follow-up test), false positives (healthy individuals incorrectly flagged as sick) can lead to unnecessary stress and expenses, making precision a priority.
Information retrieval and search engines:
- When ranking search results, precision ensures that the most relevant results appear at the top. False positives (irrelevant results) degrade the user experience.
Recall
Recall (also known as sensitivity or true positive rate) is another crucial metric to evaluate models. It’s used to measure thee ability to capture all positive classes and is calculated as a ratio of correct positive predictions to all actual positives in the dataset.
Unlike precision, which focuses on the correctness of positive predictions, recall emphasizes identifying as many true positives as possible, even if it means tolerating some false positives. This makes recall particularly relevant in cases where missing true positives (false negatives) is more detrimental than predicting false positives.
Let’s recall (another pun) our previous example: we identified 100 items as needles, out of which 90 were actual needles (true positive), and 10 were straws (false positive). Precision of this model was 90%. But with a total of 200 needles, we missed 110 of them, so our recall value sits at 45%. This model isn’t good at identifying all positive classes.
However, if we identified 380 items as needles, and 190 of them were actual needles, our model has a recall value at solid 95%. But precision is only 50%. By now you should be able to see a tradeoff between the two metrics.
This is why recall is often paired with precision, especially in applications where both false positives and false negatives have significant consequences.
At Yespo we use recall as a baseline metric for our model evaluation.
When it comes to customer segmentation, the cost of misidentifying someone as a customer is negligent.
At the same time, missing potential buyers in your campaigns can lead to decrease of your revenue, which is highly undesirable.
Pros of using recall
Prioritizes capturing positives:
- Prioritizes capturing as many true positives as possible, which is key when false negatives are dangerous (e.g., disease detection)
- Useful for imbalanced datasets where the positive class is rare
Cons of using recall
- Ignores false positives, which can lead to many false alarms
- Not suitable when precision is paramount and false positives are problematic
When to use recall
Recall is ideal in scenarios where missing positives is more critical than reducing false positives, including:
Medical diagnostics: In cancer detection or other life-threatening diseases, a false negative (failing to identify a sick patient) can have severe consequences. Recall ensures the model captures as many true cases as possible, even if it flags some healthy patients incorrectly.
Marketing segmentation: Businesses that wish to maximize their revenue from marketing activities require high recall value to capture as many potential buyers as possible. In this application, false positives (a campaign being sent to indifferent leads) have no significant negative outcome.
Disaster prediction: Predicting rare but significant events, like earthquakes or financial crashes, requires high recall to ensure critical warnings are not missed.
Recall vs. Precision
As we’ve seen, increasing the precision of a model leads to a decrease in recall, and vice versa.
Precision: Focuses on ensuring positive predictions are correct, tolerating more false negatives.
Recall: Focuses on capturing as many true positives as possible, tolerating more false positives.
Neither metric alone provides a full picture. In many cases, balancing recall and precision is critical. This can be achieved using the F1 score.
F1 Score
The F1 score is a harmonic mean of precision and recall, providing a single metric that balances both. It is particularly useful in scenarios where both high precision and recall are equally important. The F1 score ranges from 0 to 1, with higher values indicating better model performance.
Going back to our haystack examples, for the first one (90% precision and 45% recall), the F1 score will be 0.60. For the second example (50% precision and 95% recall), the F1 score will be 0.65. While the F1 scores are comparable, both models have a wildly different performance and outcomes.
When to use the F1 score
The F1 score is most valuable in cases where:
Precision and recall are both critical:
- It balances the tradeoff between false positives and false negatives.Example: movie recommendation systems, where you need to recommend relevant movies (high precision) without missing the ones that could interest the viewer (high recall).
Imbalanced datasets:
- In datasets with uneven class distributions, accuracy can be misleading, while F1 score provides a fairer assessment of performance.
Pros of using F1 score
- Balances precision and recall, providing a single metric to capture model performance
- Useful when both false positives and false negatives need to be considered
- Handles imbalanced datasets better than accuracy
Cons of using F1 score
- Doesn't allow for different weights on precision and recall based on problem context
- Can obscure insights from the individual precision and recall metrics
The F1 score is a good metric for evaluating models where precision and recall are both important and tradeoffs exist. It provides a balanced assessment, especially for skewered datasets, but should be interpreted alongside precision and recall to account for specific use-case priorities.
Predictive segmentation with Yespo CDP
Here at Yespo, we use an advanced machine learning model to segment customers based on the likelihood of purchase, the most important measure for ecommerce.
To create these predictions, our model analyzes a wide range of data, including purchase history, value and frequency of past purchases, date of the customer’s last action, patterns in behavior, demographic data, and other factors.
This approach captures and analyzes more data than any marketer can reasonably handle.
From there, our system proceeds to create a segment of likely buyers, that you can use in your campaigns.
To create a predictive segment, go to the Contacts section → Segments → Add segment → Dynamic and select the Purchase likelihood option.
You can choose one of four presets or select a manual Recall value—from 20% to 80%.
As you already know, the recall value adjusts the number of likely buyers within the created segment.
Lower values (20-50%) will create a narrower segment with a better ratio of buyers to non-buyers (since the precision will be higher). This is great for highly targeted campaigns or when your goal is to have the highest ROMI possible.
Higher values will create a broader segment that has a higher share of non-buyers (lower precision) but covers the majority of likely buyers. Use this when you need to maximize your revenue and cover a significant portion of your customer list.
When it comes to our presets, here’s what they do:
- Sure Buyers: Users with a very high likelihood of purchase. Perfect for premium products and exclusive offers, this segment can become your main revenue driver.
- Potential Buyers: Users with a medium to high likelihood of purchase. These customers respond well to seasonal sales, product launches, and personalized recommendations.
- Unlikely Buyers: Previous customers who haven't made a purchase in a long time. Re-engagement campaigns, win-back offers, and personalized messages can help return them to your business.
- Undecided Buyers: Users with a low to medium likelihood of making a purchase. They might be interested but are not yet convinced. Offering incentive-based promotions, educational content, or feedback requests can help nudge them.
Using this instrument has several major advantages:
Speed and ease of creation: Predictive segments are very simple to set up, saving you valuable time you’d otherwise spend creating complex manual groups.
Better performance: Machine learning algorithms analyze bigger amounts of data. This allows the creation of more targeted and precise segments that tend to outperform the ones created manually.
Campaign cost savings: Predictive segments excel when you send messages through channels like SMS, where the cost per message is noticeable. Using this technology, you can make every message perform and deliver cost-effective results.
New workflows: In addition to desired conversion events (e.g., purchase), predictive segments can address other events, such as customer churn. These events aren’t easy to set up with manual segmentation and allow for creative retention campaigns.
Easier A/B testing: Predictive segments tend to produce better results, making them perfect for A/B tests of different creatives, offers, and other variables.
Let’s take a look at some real-life examples of this technology.
I want to test predictive segments!
O.TAJE case study
O.TAJE, a Ukrainian women's fashion brand, dramatically improved its Viber campaigns by implementing predictive segmentation through CDP Yespo. Between June-August 2024, they tested predictive segments against their traditional manual segmentation approach.
In one notable campaign, their predictive segment achieved:
- 57.83% open rate (vs 45.24% for manual)
- 5.73% conversion rate (vs 5.14% for manual)
- 1010.89% ROMI (vs 389.19% for manual)
Overall, campaigns that used predictive segments improved the following metrics:
- 26% higher click-through rates
- 300% higher conversion rates
- 310% increase in overall ROMI
Considering the results, O.TAJE plans to use predictive segments for other marketing channels, particularly SMS.
Conclusion
That’s it—now you know everything required to effectively use predictive segmentation. How it differs from descriptive methods, what data is used, how the models are built, and how they are evaluated.
Armed with this knowledge, you can launch your own effective campaigns using the power of machine learning.
If you’d like to learn more about how predictive segmentation can work for your business—and how Yespo can help you with it—fill in the form below. Our experts will get back to you in no time.