11 June 2024
1106
8 min
5.00
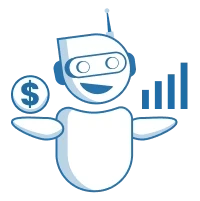
How to Save Up to 50% of Marketing Budgets With Predictive Segmentation
Marketers have relied on manual segmentation to improve their campaigns for ages. It’s a solid approach that helps handle the baseline of the problem. However, it has major pitfalls as it consumes time and provides insufficient accuracy. Let’s look at practical examples and how we can improve by applying predictive segmentation.
Manual Segmentation In Real Life
Meet Bob, a junior marketer at an ecommerce store. Bob’s task is to get the most out of the store’s customer list. The previous person made mistakes, so it’s up to Bob to make improvements.
Bob receives access to the full list with thousands of customers. Among these, there’s a person who made a purchase once 5 years ago, a customer who occasionally buys low-cost items, a buyer who makes large purchases once in a while, a VIP customer who buys a lot and often.
Bob expects that some people on the list are ready for another purchase right now. However, it won’t be relevant for the majority, no matter how good his offer or copy is.
Bob decides to start by sending out his first SMS campaign to the whole list at once. When he finally gets the results, they don’t look great. It turns out, the store has lost $926 on this campaign. If Bob wants to keep his job, he needs to ensure that this won’t happen again.
Sending messages to everyone on the list isn’t the best strategy. It may annoy people who aren’t ready to buy yet, and losses would prevail over possible gains. As in our example, it can be very expensive—to the point of loss.
Now, Bob has learned the lesson, and he chooses to narrow down the list of receivers.
He is aware that people who have made recent purchases are more likely to buy again. He focuses on the group of people who bought anything in the past 90 days. This time, the results look much better to Bob. It seems that Bob is going to keep his job.
Pay attention!
Manual segmentation has limitations that decrease its effectiveness, including:
- Obsolete Data Interference: Using outdated customer information leads to inaccurate targeting.
- Human Error: Mistakes are inevitable in manual processes, impacting segmentation accuracy.
- Scaling Difficulties: As customer lists grow, maintaining manual segmentation becomes increasingly challenging.
Now, Bob is wondering what he should do next. How should he approach his future campaigns?
How Marketers Trick Themselves Into Answering The Wrong Question
For decades, marketers have been trying to answer one question: what segmentation criteria would yield the best results? In Bob’s case, the dilemma is whether the 90-day period is the best option.
He may go further, though, and ask: what if he picks a 45-day period instead? Would it improve ROMI or make it even worse?
Should he try a different direction? Would a 120-day period still be profitable?
So many questions… and, still, the right one isn’t here. At this point, Bob should be asking himself what he should aim at. There are three main goals he may want to achieve with his campaigns:
- Maximize campaign profit.
- Maximize revenue while minimizing losses.
- Maximize reach, even if it comes with operational loss.
This is how it looks when plotted on a graph:
To avoid making guesses about segmentation criteria and start working toward particular goals, we should turn to predictive segmentation.
Predictive Segmentation as an Answer to Marketers’ Questions
Instead of splitting the customer list by the number of days since the last purchase (or frequency, or any other indirect metric), Bob would better focus on criteria that are directly tied to the desired outcomes.
The probability of purchase is the metric Bob should pick first. It helps evaluate each customer and rank them based on how likely they are to buy again after interacting with the business. However, manual methods can’t handle this; therefore, we need more powerful tools.
Predictive modeling relies on neural networks and customer data to create accurate forecasts of future behavior. Instead of operating indirect metrics, it allows us to work with the main conversion events – purchase, churn out, abandonment, subscription, etc.
Neural networks analyze huge amounts of past data to create predictions. Unlike manual methods, which often deal with limited timeframes, AI can analyze months or even years of data and find similarities between customers. This helps us identify patterns not obvious to humans, especially with large datasets.
Another metric Bob would find useful is Recall. It reflects how well the model covers all potential buyers within the chosen segment.
If your goal is to reach as many potential customers as possible, even if it includes some who aren't ready to buy, pick a higher recall value (e.g., 70-80). Alternatively, if you want to focus on a highly targeted group of likely buyers to reduce costs and improve conversion rates, set a lower recall value (e.g., 50-60).
To make this clearer, let’s look at an example: We have a list of 100,000 customers, with 1,000 predicted to be ready to buy. If we set a recall value to 80, we end up with a segment of 60,000 people. This means that 80% of our predicted buyers (800 out of 1,000) are included in this segment.
If we instead set the recall value to 55, the system generates a smaller segment with 25,000 customers. Out of these, 550 (55% of our total number of predicted buyers) are very likely to respond positively to our campaign.
Keep in mind that in the context of predictive segmentation, sensitivity works inversely proportional to accuracy. Smaller recall values result in more accurate and cost-effective segments. Generally, the recall of 80 might have 99 non-buyers for each buyer, while a recall of 55 will have only 97 non-buyers for each buyer.
Armed with a predictive model and a clear marketing goal, Bob decides to run a campaign. He aims to maximize revenue, so he sets the recall to 80.
These results turn out exactly as Bob has ever wanted them to be. His revenue is high, with no losses involved. Satisfied with the outcomes, he decides to try another approach—now, he wants his campaign to maximize business profitability. He sets the recall to 55 and sends out another campaign.
This time, Bob gains even better results. He’s sent only half the number of messages compared to the previous campaign, yet his profit is the highest of all campaigns he's run so far. With such results, Bob may not only keep his job but as well receive a promotion!
Combining all results in one picture, Bob gets the following view:
How You Can Implement Predictive Segmentation With Yespo
As we see, predictive segmentation can be a powerful tool for a marketer, allowing new levels of segmentation accuracy.
This is achieved through the deep and thorough analysis of existing data. No matter how good a marketer is, there are way too many variables for a human to take into account.
Meanwhile, machine learning algorithms can analyze enormous data and provide actionable insights.
In Conclusion
By implementing predictive segmentation in your business, you can get irreplaceable help with:
- Projecting the outcomes of your campaigns: Eliminate the guesswork and predict your results before sending the first message.
- Engaging only those customers who are ready to hear from you: Reach interested customers while reducing the irritation for those not ready to act yet, leading to better customer experience and lower unsubscription rates.
- Allocating your marketing budgets better. Spend money on campaigns that produce results and eliminate budget waste on trial-and-error activities.
Now, if you’re wondering how to start with predictive segmentation for your business, our CDP Yespo provides a convenient way to do it. Your job is to connect your data sources; the rest is on Yespo.
If you want to give it a try and see the results for yourself, fill out the form below and our team will get in touch with you.