04 September 2017
4851
9 min
3.62
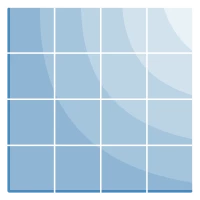
Data Science on Duty of Email Marketing Specialist
In 2012, the Harvard Business Review called the profession of a data scientist the sexiest job of the 21st century. Since all ratings of the "data science" have been constantly growing, and the demand in data scientists far exceeds the real number of specialists in this field. This trend has not bypassed the email marketing.
In this article, with specific reference, we are going to show how the data science helps email marketing specialists. In particular, it gives an opportunity to understand better the general model of customers behavior and develop fundamentally new schemes of email campaigns.
Analyzing the activity of users by the email openings, the time since the last opening, and the number of received email letters
Often, the RFM-analysis is used to analyse and segment the client base. It is based on the assumption that a customer, which:
- has recently showed some activity (recency);
- showing increased activity from the moment of registration (frequency);
- spending more money on your goods (monetary),
will be more interested in your advertising campaign. Usually, the client base is split into parts by these three metrics to run the analysis of the customer behavior models.
Although the RFM-methodology is a useful tool for analyzing an address list, one can explore other approaches that allow you to understand better your customers and to discover new, sometimes unexpected, aspects of their behavior.
Recently, for one of our clients within the specific email campaign, we carried out a joint analysis of the clients activity in the email openings, the time since the last reading, and the total number of received emails. As a result, we received the scatter plot.
Here, the abscissa is the time since the last opening, divided by the lifetime of the user within the email campaign. If a user has not opened any email, the corresponding value of the metric we define as 1. The ordinate is the frequency of opening of emails within the email campaign by the user. All users were divided into 4 categories by the number of received emails.
From the chart it can be seen the majority of users are concentrated near the origin, as well as along the axes. The group of users:
- near the origin are those who do not read much, but the last reading was recently (relative to the lifetime of a user within the email campaign);
- in the upper left corner are those who read almost everything and opened an email recently;
- in the lower right corner are those who do not read much and the last opening was a long time ago (again, in the relative values).
The remaining points correspond to users with intermediate patterns of behavior.
In the classic email marketing, it is assumed that the most influential factor that determines the activity of customers in openings and clicks is the time since the last active action (recency). To verify this statement, we have built a similar scatter plot of users activity only for those users who opened the email from the email campaign under consider.
From the chart we clearly see that the majority points are concentrated in its left half, with a predominance in the region of the y-axis. For this group of users, the linear nature of the distribution by colors is also clearly distinguished: the purple dots are closer to the axis, the blue ones are father, and so on. At the same time, the general frequency of openings does not have such a strong influence on the readings of the emails. This is primarily due to the following reasons:
- indeed, the recency factor for this (large enough) group of users has a prevalent value over the frequency one;
- among all the categories of users by the prescription of involvement in the email campaign, there are those who read the email.
But also there is a group of points of all possible colors, concentrated along the x-axis. Particularly interesting is the group of points in the lower right corner. Recall that this segment of the chart corresponds to low-activity users who opened emails rarely and for a long time. But, as we see from the chart, this concrete email letter they have read.
Let's try to define the system of rules: which users should be sent emails within the email campaign, and which are not, in order to minimize potential losses in openings and clicks.
Launching the artificial intelligence
In a number of our previous publications, we have already described the users activity filtering systems developed by us, based on the artificial intelligence (AI). Let's try to apply AI to solve the problem. Recall that we carried out the entire previous analysis in the framework of the metrics:
- relative recency;
- open rate;
- total number of received emails within the email campaign.
Based on the history of user previous activities, we developed an AI algorithm based only on these metrics. In the result of application of such algorithm to a group of users, each user will coincide with a certain number from 0 to 1 (or, alternatively, from 0% to 100%) -- the probability that this user will not read the next email within the email campaign. Choosing a particular value of probability as a threshold, we will classify users into those who should be sent the next email, and who do not (concerning the parameter "threshold" you can read in more detail here). We have chosen 0.99 as a threshold and applied our algorithm to the analysed group of users. The results are shown in the following two scatter plots.
The first chart presents the group of users to whom our algorithm did not recommend to send the email (for each of these users, it was obtained the probability at least 0.99).
The second chart shows the users who opened the email, although the algorithm recommended not to send it to them.
From the charts we can draw the conclusions:
- On the first chart, the majority of points are concentrated along the x-axis, while the values of the metric "relative recency" start at 0.55, the "open rate" of most points does not exceed 0.1. Concentration of the points near the abscissa axis indicates that the algorithm selects users with low frequencies in openings of emails. On the other hand, the range of "relative recency" (0.55; 1) means that users with a relatively long last reading of an email are selected.
- On the second chart, the points are concentrated in the stripe (0.6; 1) along the abscissa axis and (0; 0.1) along the ordinate. Distribution by colors is approximately the same.
In general, the AI algorithm developed by as recommends to exclude users with low open rate and those who haven't read lately. Limit values of the corresponding metrics are regulated by selecting the parameter "threshold". As a result, we can control both the amount of users recommended for the exclusion from the email campaign and the potential losses in openings (clicks). But from the last chart we see that even with a relatively high cut-off barrier (in our case, 0.99), the potential losses in openings will be quite significant (the number of points on the chart is not so small).
Drawing conclusions
One of the key conclusions that can be drawn from the analysis is to develop highly effective user filtering algorithms, it is not enough to use only three metrics considered above. Moreover, it is not enough to utilize only the "recency" metric as a parameter for filtering. Choosing a low value of this parameter can lead to significant potential losses in readings and clicks, whereas a high value will lead to psychological fatigue of some part of the subscribers and, as a result, to burnout of the content base.
To increase the accuracy of classification, it is necessary to include new metrics (clicks, channels, gender, age, etc.) into the algorithm that will further separate users with low values of open rate and high values of "relative recency" according to the probabilities in emails reading. That is why in the algorithms that we developed, we use several dozen of different metrics (in some cases -- up to 50-60 ones).
Next important conclusion: while traditional methods of user activity analysis (for example, the RFM analysis) require static breakdown of the client base into segments manually ("low-active", "VIP", etc.), the AI systems allow to automate this process by dynamic regulation only one parameter -- the probability of emails reading.
In our case, having chosen the threshold equal to 0.99, we found the group of low-active clients, while the choice of limit values of the metrics "open rate" and "relative recency" was made automatically. Similarly, one can select a group of VIP-clients, etc. By changing the threshold, you can additionally adjust the parameters of the analyzed segments.
You can find the best Artificial Intelligence development companies in 2019 in that article - https://thinkmobiles.com/services/artificial-intelligence/