25 February 2015
11084
8 min
3.25
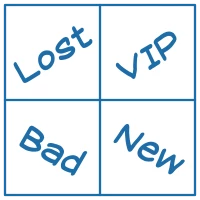
Analysis of Data in E-Marketing. Part 2: RFM Segmentation
How organic and simple is the RFM analysis in e-marketing, so it is scaringly far away for those who have not yet started using it. Personally, we liked this approach so much that we already wrote articles about it twice (one and two) and even integrated it into our email marketing system (now the RFM module is in the beta stage and is available to system users on request).
And here we are again writing about RFM, however, this time we will show how it is built on a concrete example, using the database of the fictitious online store “Tip-Tops”. We will try to explain everything in detail and human language, so we invite to reading and experimenting marketers, for whom RFM is so far only a familiar abbreviation, and not a practical tool.
Spreadsheets Analysis_Sample
A file with a database and all calculations can be downloaded from the link. We have already described this file and working with it in the first article of the analytical series - Data Analysis in E-Marketing. Article 1 - Channels for Attracting Customers - so here we will dwell only on what is needed directly for RFM analysis.
And we need only the sales base, which contains information about date, order price and customer id. The results we find on the “RFM_Analysis” sheet, while part of the interim calculations is automatically done on the “Customer_Base” sheet.
RFM Analysis — It's Simple
What is RFM-analysis? It, only, one of ways of segmentation of base of customers.
By what principle is it segmented? According to customer's activity. We watch how often he made purchases (Frequency), how long (Recency) and how expensive (Monetary). Have not made purchases yet? Also not a problem.
What will it give us? We can “approximate” our marketing proposal to customer, without even knowing any additional information about him.
Where does it work? Wherever there are many customers and opportunity to address each one personally. We analyze frequency and recency of purchases in ecommerce, but with the same success, it can be reading letters in subscription business or calling in application in SaaS.
At the very beginning, I already provided RFM-analysis with epithets “organic” and “simple”. This is, indeed, the most natural way to get an idea about customer without additional information about him. All that is needed is the story of our relationship with customer. And in some cases RFM can be simplified to RF, i.e., analysis of prices can be sacrificed for the sake of greater simplicity and universality. Fortunately information about frequency of purchases can indirectly, but with great certainty, say about income that customer brought us.
Next, we show how we segmented our sales base by R, F and M.
Customer Base
The first thing we did was build a database of customers with fields for each one as follows:
- customer id (unique identifier)
- last order date
- number of orders
- amount of prices for all orders
We did not need the help of a database programmer to do this, all the data we already had in the sales database. It took, of course, some knowledge of formulas of spread sheet editor, although the same result could be obtained with the help of PivotTable tool, which is more known than the formulas for working with arrays.
The main goal was to form a table where each customer has his own line and can be met only once, unlike the sales base, where he could be met as many times as orders he made.
Recency
For segmentation by Recency, i.e., by the recency of last purchase, we set periods for four segments. Please note, all user data in our file is entered in orange cells.
We chose:
- Segment 1 - Up to a month
- Segment 2 — Up to 3 months
- Segment 3 — Up to a year
- Segment 4 — More than a year
The most favorable segment for us - the segment of the most recent purchases – it has number 1. If you are used to reverse numbering, you can change the order in orange cells of the last column of the table shown above.
The last date of our entire database is known, i.e., we can count from it in the reverse direction of the duration of segments and get the dates of their boundaries.
And now we need to compare the date of boundaries of segments and the date of last orders and already for these dates assign numbers of segments 1-4 to each customer.
The result is in the “Recency_Segment” column on the “Customer_Base” sheet. The total number of customers by segments can be seen in the screenshot above from the “RFM_Analysis” sheet.
Frequency
Similarly, we set boundaries of segments, this time - number of orders:
- Segment 1 — 1 order
- Segment 2 — 2 orders
- Segment 3 — 3-4 orders
- Segment 4 — 5 orders and more
The result can be seen in the “Frequency_Segment” column on the “Customer_Base” sheet and in the corresponding table on the “RFM_Analysis” sheet.
Please note that in our database are only customers who made at least one purchase. The same analysis can be done with the participation of customers who did not make purchases at all, but this situation is not processed in our formulas and requires a small adjustment.
You also have to adjust the calculation and increase the number of segments. We chose 4 segments for each direction, so as not to engender a myriad of options. After all, it is assumed that each segment will be tied to some kind of marketing action.
Monetary
As already mentioned, interesting and useful results can be obtained already with the joint analysis of R and F alone. We have also built segments for M, there everything is similar to the previous two directions. The result is in the “Money_Segment” column on the “Customer_Base” sheet and in the corresponding table on the “RFM_Analysis” sheet..
RF Matrix
As a result of our analysis of R and F, we build a matrix, placing Recency segments horizontally, and Frequency segments vertically. We have 16 (4x4) derived segments of customer base. This is already quite a lot, since we need to configure the appropriate response for each customer segment. But the whole point of matrix matching of R and F is that we can merge segments, allocating groups of customers at our discretion.
Someone suggests combining all small segments into four quadrants (for example, Jim Novo in the article on his blog), someone - using a personalized matrix partitioning.
We chose the second path, defining 7 customer segments. This allowed us to reduce from 16 to 7 the number of groups for which we prescribe individual actions. The result is shown in the screenshot above. In the first table of the screenshot, the number of customers from our database in each segment is counted, in the second one - the segment is decoded, in the third - examples of marketing actions for each segment.
To Be Continued…
Please note that we have identified a segment of those customers who have not purchased for a very long time - the “Departed” segment. We can rightly delete from the list of active customers, thus saving on personal marketing for them.
In addition, the “Departed” segment can set us a criterion for the end of the customer's life cycle, which is so ambiguous in ecommerce. We will show how this can work to calculate churn of customers and the Lifetime value by the example of cohort segmentation, which we will talk about in the next article of our analytical series.
Stay tuned :)
P.S. RFM analysis, as we have already said, is very effective in email marketing, as it allows to fully automate “smart” personalized marketing mailings. We are working to ensure that such an opportunity appeared in the our system. There is a beta version of the visual segmentation of recipients of mailings by reading already available for the users. In our plans - adding possibility of analyzing sales data for ecommerce and setting up automatic mailing scenarios by RFM segments.
Analysis of data in e-marketing. Article 1 - Channels for attracting customers
Analysis of data in e-marketing. Article 3 - cohort analysis and LTV